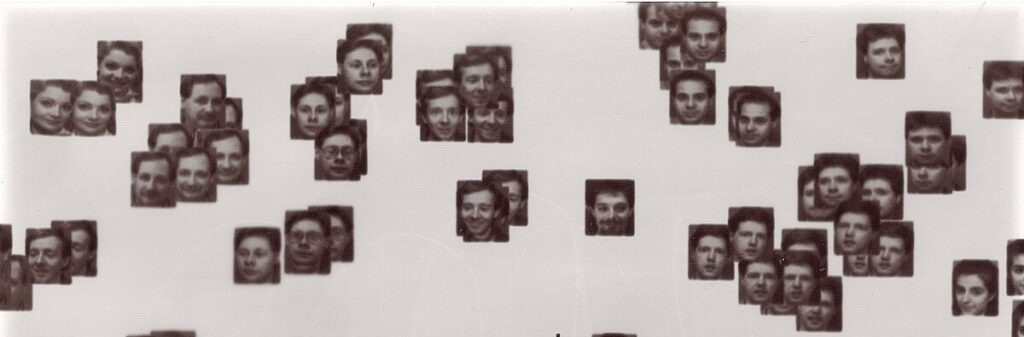
Philipp Schmitt & AT&T Laboratories Cambridge / Better Images of AI / Data flock (faces) / CC-BY 4.0
As AI technology spreads into more areas of life, making sure it is fair and unbiased has become much more important. However, we can’t assume AI systems will automatically be fair – bias can easily creep in if we aren’t careful.
If unfair bias builds up in AI systems, it can amplify social unfairness and hurt vulnerable groups even more. Even developers trying their best often miss biases shaping algorithms.
Before looking at the harms bias causes, we will explore how bias occurs during the process of building AI tools – from collecting data to real world testing. Explore how bias can occur in this interactive diagram:
Here are some images that have been generated by Bing Chat using the prompt “create an image of a mancunian dog”.
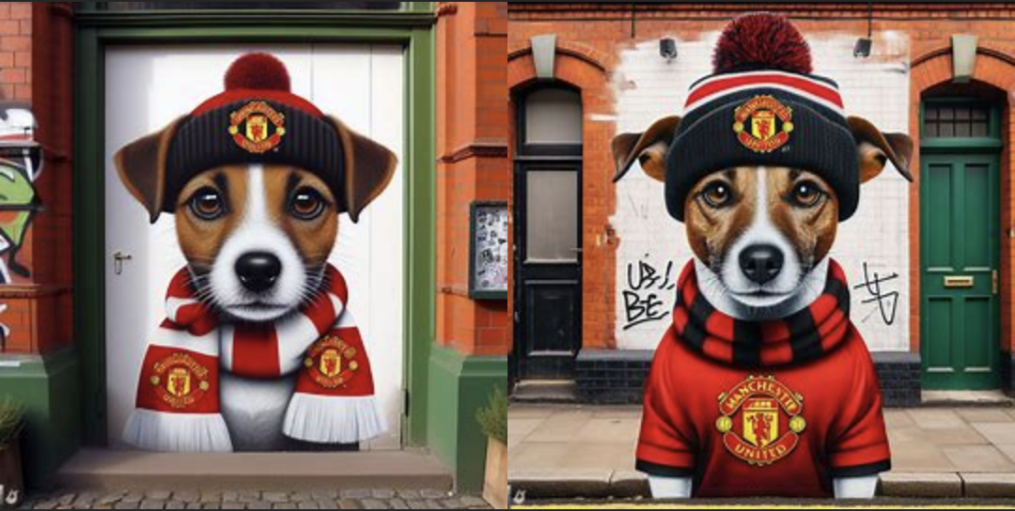

Stop and Reflect:
Here are some images that have been generated by Bing Chat using the prompt “create an image of a mancunian dog”.
Think about your experience of Manchester and consider how representative of Manchester these images are.
- Do they accurately present what a mancunian dog would look like?
- Where might the bias shown in the images have come from?
- How might the wording of the prompt have influenced the types of images that have been generated?
The bias shown in the Mancunian dog images is obvious, but that isn’t always the case. The following videos give examples of how bias can manifest in AI tools and the impact that they can.

Watch: Algorithms of Oppression: Safiya Umoja Noble
In this video Safiya Umoja Noble highlights how biased search algorithms can reinforce racism, sexism, and oppression. Through specific examples and her own research, Sofia makes the case that search engines reflect the values and biases of their creators – with serious consequences for marginalised groups. As we watch, consider the ethical dimensions around search algorithms and knowledge platforms:
- Who benefits from the status quo?
- Whose voices and perspectives get amplified or suppressed?
- What kinds of alternatives does Sofia propose?

Watch: Gender Shades
In this video, Joy Buolamwini underscores the real-world impacts biased systems can have and argues we have an ethical responsibility to address these issues. Watch the video and consider the following questions:
- What surprised you the most from Joy’s presentation? Were there any key ideas or statistics that stood out to you?
- Joy argues that there is a lack of diversity in the training data used for facial analysis systems. Why do you think this matters? What impacts could this have?
- The issues highlighted in Joy’s talk point to larger concerns about bias in artificial intelligence systems. In your opinion, what is the responsibility of tech companies when it comes to addressing these issues? What should they be doing?