Parametric Assumption 1 – Normality
When conducting bivariate or multivariate tests, to check our data is normally distributed, we use Q-Q plots. We can run Q-Q plots for normal distribution and then run a Levene’s Test for homogeneity.
In SPSS we can run Q-Q plots and Levene’s at the same time.
Select Analyze > Descriptive Statistics > Explore. A box will open as shown below.
If we wanted to test the hypothesis: There will be a gender difference in Life Satisfaction in Great Britain, we would move the dependant variable LifeSat into the Dependent List box and the independent variable Gender into the Factor List.
Then choose Plots. In the box that opens, under Descriptive untick stem and leaf and tick Histogram. Under Boxplots, tick None. Tick Normality plots with tests. Finally, under Spread vs Level with Levene Test, tick Untransformed. Click Continue and then OK.
Several different outputs will be produced.
Interpreting Q-Q Plots for normality.
SPSS produces two types of Q-Q plots, normal and detrended but we’re only interested in the normal ones. Additionally, SPSS produces Q-Q plots for each independent variable so each need to be reviewed before one can claim the data is normal (or not). In this example we’re using gender, a dichotomous variable with only two groups within it, we therefore only need to review two Q-Q plot outputs. The figures below show the Q-Q plots for our data.
Data is deemed approximately normally distributed if the dots follow the line (although they don’t have to sit on the line).
Data would be deemed not-normal if it formed an approximate S-shape.
Reviewing our Q-Q plots above we can see that our data is approximately normal because it follows the line, we can see for both Males and Females there is a slight tail or skew to the left, but it is not great.
Interpreting Q-Q plots gets easier with time and sometimes it is just up to your own judgement, if you clean your data properly prior to assumption testing the removal of outliers should make it easier to establish normality.
Below are two examples of S shaped Q-Q plots for reference, these would be deemed not-normal, and would violate the parametric assumption for normality.
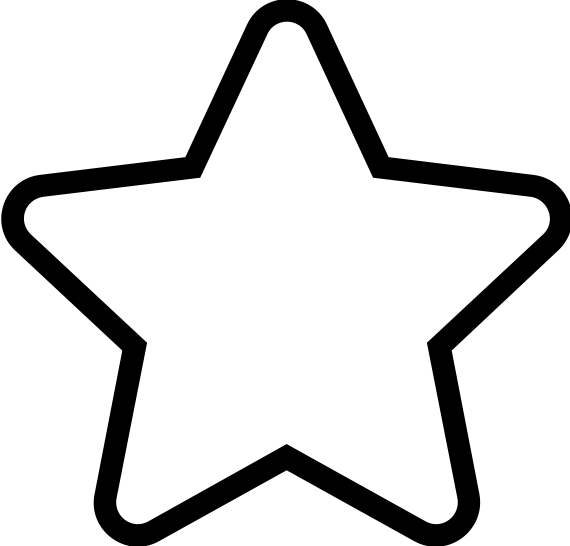
Apply Your Thinking:
Review the outputs below and present your findings for the following assumption testing
Write your findings down on a separate word document
Review the outputs below and present your findings for the following assumption testing.
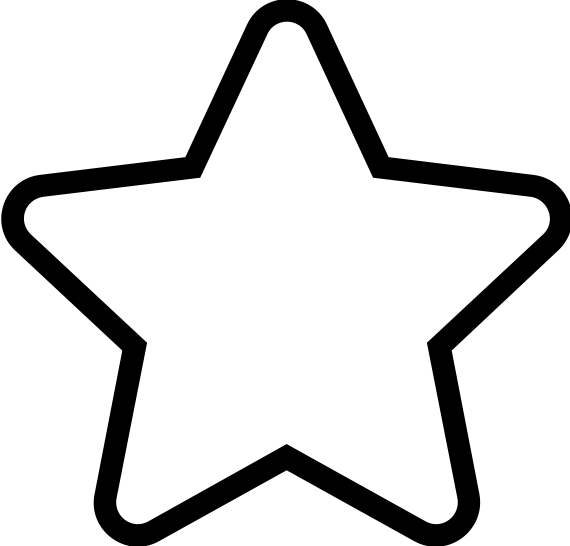
Apply Your Thinking:
<strong>Now</strong> complete parametric assumption testing for the hypothesis: <strong><em>Life Satisfaction will differ according to ethnicity in Great Britain.</em></strong>
Perform a normal distribution test in SPSS.